Purpose in short: I’ve got both illumina (PE and MPE) and PacBio reads of an insect for de novo geome assembly. Since whole bodies were used for DNA extraction, I thought there was some contamination in the raw sequencing reads, so I want to remove them before assembling.
The main tool for illumina reads I used was BBDuk
, which belongs to the BBTools suite. Seal
and BBSplit
can also do this, but BBDuk
is the most suitable for my purpose. See discussions here: Question: How to remove contamination from the transcriptome assembly. BBTools
is quite versatile, fast, and convenient! Though now I don’t fully understand every kit in it, the experience was very good from the tools I’ve tried. The author Brian Bushnell is very active and responsive. Here is a handy summary of BBMap
: Yes … BBMap can do that!.
But for PacBio long reads, I didn’t find any tools specialized for that. I tried several tools including mapPacBio.sh
(based on bbmap
, and is tuned for the error profile of long reads.), blasr
, MashMap
, and minimap2
. At last, I found minimap2
best met my needs.
Prepare the contamination library
-
Download all the sequences of bacteria, viral, fungi, protozoa, and archaea using refseq2kraken. See note Detect Microbial Contamination in Contigs by Kraken for details. We can also use ncbi-genome-download to download them.
1
2
3folder structures
ls genomes/refseq/
archaea bacteria fungi protozoa viral -
Merge all the sequences into one single Fasta.
-
Since I’ve got the mitochondrion DNA sequences of the target organism, I added them in the contaminant library to remove reads from mtDNA too. This library is refered as to
$contaminants
below. -
After discussing with the author of
MashMap
, I included several insects’ genomes of the same order of my target insect to improve the specificity of aligners. This library is refered as to$contaminants2
.
BBDuk for short reads
BBDuk is a member of the BBTools package. “Duk” stands for Decontamination Using Kmers. BBDuk is extremely fast, scalable, and memory-efficient, while maintaining greater sensitivity and specificity than other tools.
1 | WORKDIR=/parastor300/niuyw/Project/beetle_genome_171231/data/second |
The ‘good’ reads will be in ${sample}.R*.fq
, and the ‘bad’ ones will be in ${sample}.bad.R*.fq
.
Long reads
mapPacBio
mapPacBio.sh is based on bbmap
, and is tuned for the error profile of long reads.
1 | WORKDIR=/parastor300/niuyw/Project/beetle_genome_171231 |
The output of bbmap
or mapPacBio.sh
is SAM
, and both mapped and unmapped reads are saved in one file. reformat.sh
was used to extract mapped reads and transfrom it to FASTA
. See this: Question: bbmap command to extract mapped and unmapped pair end reads.
But mapPacBio
was very very very slow, even I used 24 threads. After about 25 days, the ouput was about 4.6G (my input was about 60G!), though it was still increasing, I killed the job.
blasr
blasr may be the one of the first long-read aligner [1]. So I gave it a shot.
1 | TOOLDIR/anaconda2/bin/blasr third_all.fasta $contaminants --nproc $PPN --out blasr.bad --unaligned blasr.good |
Related: output unmapped reads.
But it went wrong:
1 | [INFO] 2018-07-02T18:56:18 [blasr] started. |
What?! My fasta was about 60G. If I wanted use blasr
, I had to split the input into small ones, but I didn’t want to.
MashMap
MashMap is a fast approximate aligner for long DNA sequences [2]. It’s very fast and is easy to use.
1 | PPN=8 |
-s
is the minimum query length (default is 5000), and --pi
is the minimum identity to be reported (default is 85).
The output of MashMap
is like this. Separated by space, it is query name, length, 0-based start, end, strand, target name, length, start, end and mapping nucleotide identity
in turn.
1 | m161109_080520_42256_c101052872550000001823247601061737_s1_p0/104/24935_31494 6559 0 4999 - NC_037282.1 2038340 596479 601478 82.1711 |
Here is also a thread talking about contamination: Decontamination of bacterial sequences in an assembly. The author suggested that “One more thing that may be helpful for you is to include the representative genome (corresponding to your assembly) in the database as well. This would help improve the specificity of the method for correct portions of your assembly.”
But, the default parameters MashMap
use are a bit loose, I wanted to use more strict parameters. When I lowered the -s
and --pi
, the program needed huge RAM and couldn’t run on our machine.
I’ve reported this issue to the author: Decontamination of bacterial sequences in an assembly.
And, there were also some questions about its alignment: Questions about the alignment of MashMap.
I first ran MashMap
with different parameters and got several outputs:
1 | run1, with default parameters |
And the outputs from three runs varied:
1 | there are 6633142 sequences of input |
As can be seen, nearly all the sequences were aligned to contaminant library. That really shocked me!
Then I checked the top 10 sequences with highest identity and top 10 ones with loweset identity from the first run using blastn
. The highest ones were fine. There were some differences between hits reported by blastn and MashMap, but maybe it’s because they used different databases. But the loweset ones were problematic. Most of them were ‘No significant similarity found’ when default parameters of blastn
were used. And when I unselected ‘Low complexity regions’, the alignments were unreliable. There maybe something with ‘low complexity regions’ or ‘repeat’ things.
And the author explained:
Mashmap identity is an estimate based on Jaccard similarity- not the precise identity; unfortunately the Jaccard-similarity based metric delivers poor specificity in cases when the source of reads is absent from database. See if you can include an insect reference genome (s) in the reference list to avoid this.
Then I added several insects’ genomes of the same order of the target insect into the contaminant library and ran MapshMap
again:
1 | path2mashmap -t 20 -r $contaminants2 -q third_all.fasta -s 500 --pi 80 -o mashmap4.out |
For each read, it will be one of two states: mapped or unmapped, and for the mapped, it will be one of three states: mapped to insects (good), mapped to contaminants (bad) and mapped to both (ambivalent). So I counted reads in each categories:
1 | No. of total reads: 6633142 |
As can be seen, majority of reads had been mapped ambivalently, and here is a example of such reads:
1 | m161123_064622_42256_c101049952550000001823247601061783_s1_p0/52396/4594_8610 4016 3500 4015 + NW_017852934.1 2683736 1681820 1682319 79.4204 |
So it’s very hard to extract good ones.
After all this, I got two points:
- I should add some insects’ genomes into the library to reduce the false positive hits.
- Alignment from
MashMap
maybe not so reliable ().
minimap2
minimap2 is a versatile pairwise aligner for genomic and spliced nucleotide sequences created by Heng Li [3]. It’s easy to use and runs very fast.
mimimap2
1 | PPN=24 |
I had about 60G input, and minimap2
was so fast, finished after 77 CPU hours (4 real hours, 24 threads).
The output was very huge (~685G!), and it’s like this (truncated, and the sequences were replaced by ‘seq’.):
1 | m54174_171023_074758/9962478/22561_26454 4 * 0 0 * * 0 0 seq * |
We can clearly found that the same sequence had been mapped to different reference sequences, and it’s been reported several times, with the exactly same line content.
This is a limitation of minimap2
, as reported: Multiple empty hits? and How does using a multi part index affect the accuracy?. Specifically, when a huge reference is used, minimap2
will split it into multiple parts and align all queries against each part independently. For most parts, minimap2
will print unmapped records.
The good news is that Minimap2-2.12 (r827) had addressed this bug.
minimap2-arm
minimap2-arm is a solution provieded by Hasindu Gamaarachchi, and it merges the results from a multi-part index to achieve a considerably similar output from a single-part index. So I cloned this modified minimap2
to run the job anain.
1 | install |
I counted reads in each categories like I did in MashMap
part:
1 | No. of total reads: 6633142 |
Then I kept the ‘unmapped’, ‘good’ and ‘ambivalent’ reads for downstream analysis.
To parse the result and get the clean sequences, I used a simple python script to extract clean fasta and bad fasta ids. (appendix 1)
To validate the effciency, I checked several sequences by blastn
manually.
Belows are two examples.
This is the alignment of ‘m161109_080520_42256_c101052872550000001823247601061737_s1_p0/28/0_4873’ by minimap2
.
1 | grep 'm161109_080520_42256_c101052872550000001823247601061737_s1_p0/28/0_4873' minimap2.sam |
This is the results of blastn
, and I marked them with taxonomy ID. The results agreed well with that of minimap2
.
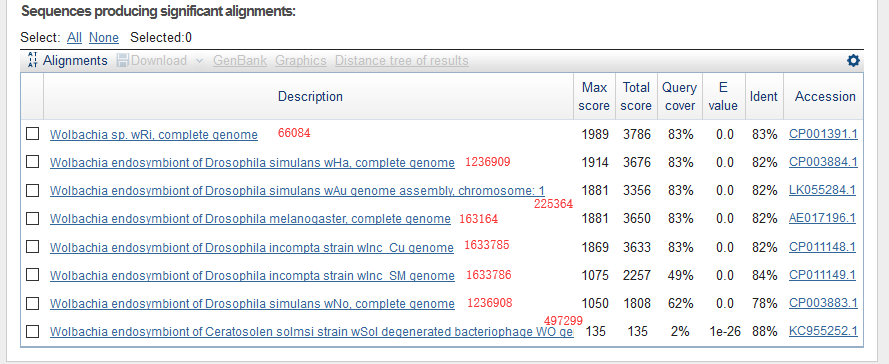
Here is another example.
1 | grep 'm161109_080520_42256_c101052872550000001823247601061737_s1_p0/796/0_3902' minimap2.sam |
And, I got different results using blastn
.
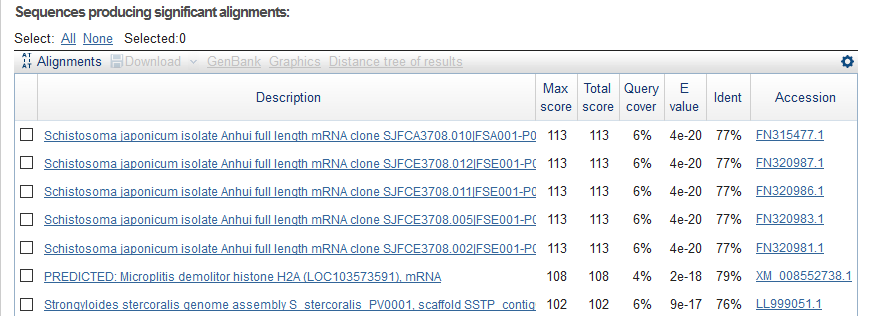
And the detailed alignment of the top 1.
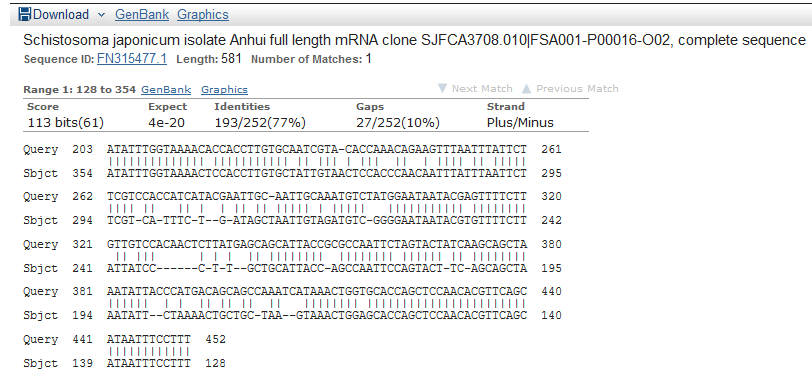
But when I checked the raw sequence, there are lots of repeats. I don’t know what it is.
1 | m161109_080520_42256_c101052872550000001823247601061737_s1_p0/796/0_3902 RQ=0.860 |
The differences between minimap2
and blastn
are explainable, they used different algorithm and different databases. And generally, I think minimap2
is reliable.
In summary
In summary, I removed contaminants in reads by the following steps:
- Prepare contamination library (bacteria, viral, fungi, protozoa, and archaea from Refseq and mtDNAs).
- Use
BBDuk
to remove contaminants from illumina short reads. - Use
minimap2
to remove contaminants from PacBio long reads.
But there are some concerns existing:
- Some real sequences may also be removed along with the contaminants.
- Many repeat sequences were removed, and I don’t know where they were from.
- For the ‘ambivalent’ reads, I kept them for downstream analysis, but I didn’t know whether they should be removed, say, throw away reads which the primary aligment were contaminant.
There are some other long-reads mapper, and people also try to tune the parameters of short-read aligners to work with long-reads. There are some threads/posts talking about this:
- Question: Long read alignment
- Question: Long read-alignment + variant calling
- Question: Alternative to BLASR ?
- Question: BWA-MEM using long PacBio reads
- Mapping long reads with Bowtie
- STAR: segmentation fault when using long reads
I don’t really understand the “mapping” things now, but I expect that there will be several dominant tools for long-reads mapping, just as short-reads mapping.
Useful links
- Question: Removing contaminations from PacBio reads
- How can I download RefSeq data for all complete bacterial genomes?
- Introducing BBSplit: Read Binning Tool for Metagenomes and Contaminated Libraries
- Yes … BBMap can do that!
- From raw reads to assembly STEP by STEP
- Removing contamination with BBDUK
- Question: Tool to separate human and mouse rna seq reads
Change log
- 20180628: create the note.
- 20180725: complete the note.
- 20180807: update the ‘minimap-arm’ part, add the results of
kraken
. - 20180815: add the part of ‘Minimap2-2.12 (r827)’
Chaisson MJ, Tesler G. 2012. Mapping single molecule sequencing reads using basic local alignment with successive refinement (BLASR): application and theory. BMC Bioinformatics. 13:238. doi:10.1186/1471-2105-13-238. ↩︎
Jain C, Dilthey A, Koren S, Aluru S, Phillippy AM. 2018 Apr 30. A Fast Approximate Algorithm for Mapping Long Reads to Large Reference Databases. Journal of Computational Biology. doi:10.1089/cmb.2018.0036. [accessed 2018 Jul 2]. https://www.liebertpub.com/doi/10.1089/cmb.2018.0036. ↩︎
Li H. 2017 Aug 4. Minimap2: versatile pairwise alignment for nucleotide sequences. arXiv:170801492 [q-bio]. [accessed 2018 Jan 10]. http://arxiv.org/abs/1708.01492. ↩︎